Detecting different fault locations on a bearing
Detecting and monitoring operational modes in a machine is a very effective way of getting a precise view of the asset’s health conditions. In a machine, different operational modes can reflect different usages, a production process, an on-off state, or a developing failure.
But besides reflecting the overall state of the machine, Mode Identification can also be used to detect specific conditions, like different load conditions or fault locations on a bearing.
Here, we are going to use the popular Bearing Vibration Data Set from Case Western Reserve University as a benchmark to demonstrate how different bearing conditions and faults can be properly correlated to a different operational mode, and ultimately to the automatic identification of healthy and faulty operational conditions.
Background
Data originates from a test-rig experiment consisting of a motor, a torque transducer, and a dynamometer. The tested ball bearings are mounted on the motor shaft and the data are vibration signals recorded from the drive end of the motor. The vibration signals are recorded with a sampling rate of 12 kHz. The bearings were subjected to loads in the range 0 hp to 3 hp, resulting in rotational speeds in the range of 1800 and 1730 rpm. The faults were manually introduced at the inner raceway, outer raceway, and balls.
Identification of operational modes
MultiViz Vibration’s Mode Identification feature is powered by our Automatic Mode Identification (AMI) unsupervised algorithm for multivariate time series analysis. It performs multidimensional data segmentation and clustering in time series data, such as waveform vibration signals. It detects time periods in which the data exhibits a similar behavior and reports these periods as belonging to the same operational mode.
Operational modes are often correlated with typical conditions of an asset, like on/off, load conditions or fault states. Thus, the identification of different modes when the behavior of the machine has remained the same, can point to the appearance of a fault in the machine.
To perform Mode Identification with the MultiViz Analytics Engine, the user simply needs to upload vibration time series data and the asset’s identifier. Then, just needs to make a request to the API using the Python package, the mode identification analysis.
Results
The first experiment had the MultiViz Analytics Engine detecting different loads conditions of a machine operating in a healthy state. The machine was subjected to four different load conditions, mixed over time, which in turn was affecting its rotational speed. Mode identification was able to identify these four different load conditions as four different modes and the transitions between these conditions. The image shows how each color represents a different load condition.
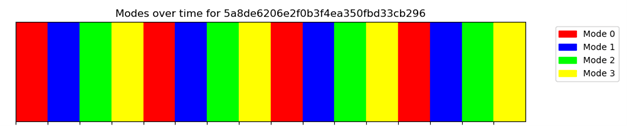
The second experiment had the MultiViz Analytics Engine identifying one healthy condition from three different fault conditions. These fault conditions were damage to the bearing in the inner raceway, outer raceway, and balls. In addition, all these health conditions were subjected to different loads randomly.
AMI was able to recognize these health/fault conditions as four different modes. Furthermore, even though there were different loads as well, it was able to identify the larger strength of the fault component within the vibration signal and as such to classify the different modes according to the health condition and by the load condition. The image shows how each color represents a different health condition.

If you collect vibration data from machine and would like to use it to detect failures and abnormal operational modes, click here to learn more about MultiViz Vibration and request a trial.